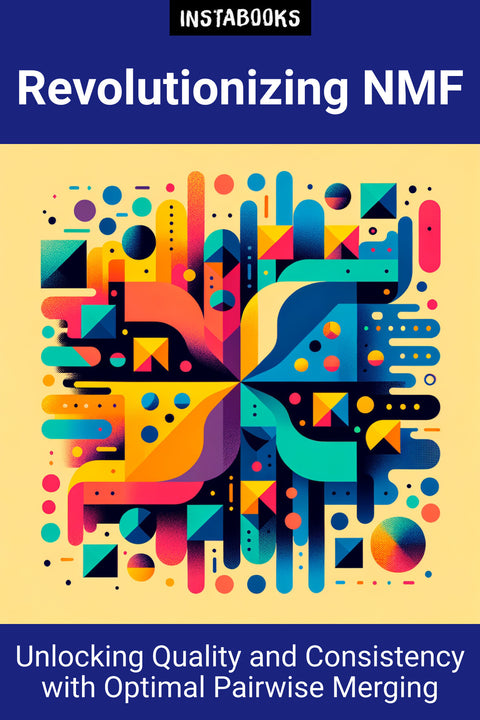
Instabooks AI (AI Author)
Revolutionizing NMF
Unlocking Quality and Consistency with Optimal Pairwise Merging
Premium AI Book (PDF/ePub) - 200+ pages
Introduction
Welcome to a groundbreaking exploration of nonnegative matrix factorization (NMF) through the lens of an innovative algorithm: the optimal pairwise merge. This book offers a deep dive into improving NMF's quality and consistency by tackling traditional limitations head-on. You will embark on a journey that unveils how the smartest algorithms are redefining data analysis in domains like image processing, text mining, and beyond.
Understanding Traditional Limitations
NMF has been a powerful tool in data analysis, yet its effectiveness can be hindered by convergence to poor local minima and challenges in component selection. This book addresses these longstanding issues, offering a fresh perspective on overcoming these obstacles through the optimal pairwise merge algorithm, designed to enhance the quality of the results.
The Power of Optimal Pairwise Merging
The heart of this book lies in the optimal pairwise merge algorithm—an ingenious method that merges components analytically to improve consistency and accuracy. By operating in a higher-dimensional feature space, it reduces convergence issues and ensures effective component merging through a linear combination of original components, tested through an objective function.
Practical Implementations and Efficiency
Learn how to implement NMF effectively with practical techniques that incorporate the optimal pairwise merge strategy. This section covers analytical solutions to normalisation constraints and enhances computational proficiency by bypassing convergence stalling. Readers will delve into practical applications across various data types, using concrete examples and scenarios.
Real-World Applications
Discover the versatility of the optimal pairwise merge algorithm in real-world applications like image processing, improving feature extraction, document clustering, and topic modeling in text mining. Additionally, explore its broader applications in speech recognition, video summarization, and more, showcasing its vital role in modern computational solutions.
Conclusion
This book merges theory with practical understanding, empowering you with detailed insights and real-world techniques to harness the full potential of nonnegative matrix factorization with optimal pairwise merging.
Table of Contents
1. Introducing Nonnegative Matrix Factorization- History and Evolution
- Core Principles of NMF
- Current Challenges in NMF
2. Traditional Limitations and Challenges
- Convergence Issues
- Component Selection Dilemma
- Resolving Local Minima Problems
3. The Optimal Pairwise Merge Algorithm
- Concept and Development
- Key Features and Benefits
- Comparative Advantages
4. Mathematical Foundations
- Higher-Dimensional Feature Spaces
- Objective Function Design
- Analytical Solution Techniques
5. Implementing the Algorithm
- Step-by-Step Guide
- Useful Tools and Resources
- Troubleshooting Tips
6. Efficiency and Performance Improvements
- Reducing Convergence Stalling
- Handling Normalization Constraints
- Computational Proficiency
7. Applications in Image Processing
- Feature Extraction Techniques
- Enhancing Image Quality
- Case Studies and Examples
8. Text Mining and Beyond
- Document Clustering Applications
- Improving Topic Modeling
- Broader Data Analysis Uses
9. Expanding The Horizons
- Speech Recognition Uses
- Source Separation Techniques
- Video Summarization
10. Future Trends in NMF
- Research Directions
- Technological Innovations
- Potential Challenges
11. Integrating With Other Methods
- Combination with Machine Learning
- Hybrid Approaches
- Cross-Disciplinary Applications
12. Conclusion and Final Thoughts
- Reflecting on Key Insights
- Potential for Future Developments
- Resources for Further Learning
Target Audience
This book is intended for researchers, data scientists, and advanced practitioners looking to deepen their understanding of nonnegative matrix factorization and its applications.
Key Takeaways
- Gain a comprehensive understanding of the limitations and challenges in traditional nonnegative matrix factorization (NMF).
- Learn about the optimal pairwise merge algorithm and its impact on improving consistency and quality within NMF.
- Explore practical implementation techniques and real-world applications across various domains.
- Understand the mathematical foundations and computational efficiencies gained through this advanced algorithm.
- Discover future trends and integration possibilities with other computational methods.
How This Book Was Generated
This book is the result of our advanced AI text generator, meticulously crafted to deliver not just information but meaningful insights. By leveraging our AI book generator, cutting-edge models, and real-time research, we ensure each page reflects the most current and reliable knowledge. Our AI processes vast data with unmatched precision, producing over 200 pages of coherent, authoritative content. This isn’t just a collection of facts—it’s a thoughtfully crafted narrative, shaped by our technology, that engages the mind and resonates with the reader, offering a deep, trustworthy exploration of the subject.
Satisfaction Guaranteed: Try It Risk-Free
We invite you to try it out for yourself, backed by our no-questions-asked money-back guarantee. If you're not completely satisfied, we'll refund your purchase—no strings attached.